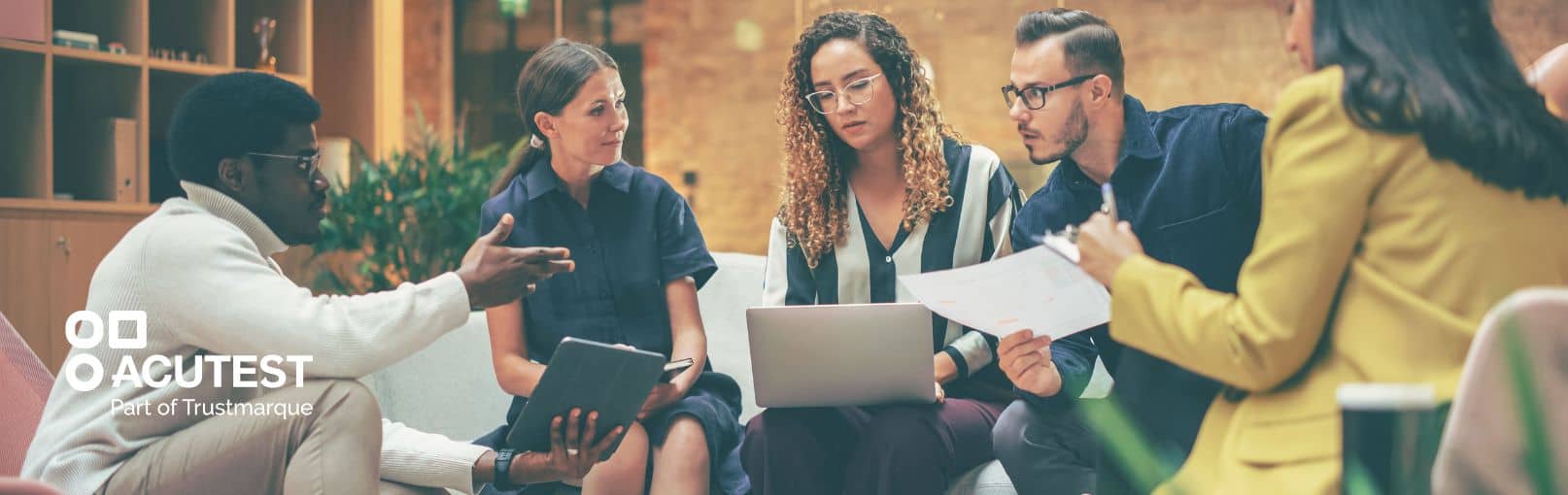
Quality Assurance in Big Data and Data Platforms projects
In today’s digital world, data is everywhere and growing fast. We are constantly generating data. Every time we post on social media, shop online, or use smart devices, we create data.
This article explores big data and how to make sense of it with advanced technologies, such as multi-layered data processing and storage solutions, and how we conduct testing in big data projects to guarantee quality.
What is it?
Big data is nothing new. Investopedia defines it as large volumes of information being analysed to reveal patterns, trends, and connections, such as those related to human behaviour and interactions, market trends, and operational efficiencies.
According to Google Cloud, it holds valuable insights that can drive innovation and efficiency across many industries. By analysing this information, businesses can uncover previously hidden patterns and trends.
AI and machine learning are particularly useful in this process, as they can automate the detection of complex patterns and provide predictive insights. For example, AI can analyse customer information to predict which products will soon be popular.
Additionally, AI can segment customers based on purchasing behaviour, helping businesses adjust their marketing strategies more effectively.
The insights gained from big data analysis can lead to significant competitive advantages and drive business growth.
Specifically, businesses can leverage this information to make better decisions quickly, improve customer satisfaction by offering personalised services, and enhance operational efficiency by identifying and resolving issues faster.
What is a data platform?
We need advanced technologies and techniques to make sense of all this information, which is where a data platform comes in.
According to MongoDB, a data platform is an integrated set of technologies that collectively meet an organisation’s end-to-end data needs. It enables information acquisition, storage, preparation, delivery, and governance.
Essentially, it acts as a central hub where all data-related activities happen, ensuring data is accessible, secure, and well-governed.
One key concept within these platforms is the medallion architecture. This architecture often organises data into layers:
Landing layer: This is the initial layer where information is ingested and temporarily stored before being processed.
Bronze layer: This is where raw and unprocessed information is stored.
Silver layer: This is where the information is cleaned and processed.
Gold layer: This layer contains high-quality information that is ready for analysis. It’s the most valuable data, used for generating insights and making decisions.
Overall, this structured approach improves data quality and accessibility, making it easier to manage and analyse.
But what about Quality Assurance (QA)?
Specifically, what role does quality assurance play in such projects? How can QA contribute to the overall success and efficiency of these projects?
The Role of Quality Assurance: why does it matter?
Maintaining quality at every stage of development is crucial for these platform projects.
To maintain this quality, begin by defining clear project requirements and scope. Next, review and approve the data platform architecture, before specifying quality checks across all layers (Landing, Bronze, Silver, Gold). Lastly, maintain high standards in reporting.
Best practices in Quality Assurance for data platforms
To ensure the success of a data platform project, it’s essential to follow best practices.
These practices begin with defining clear project requirements and scope and extend through architecture design, testing various medallion layers, and reporting.
The best practices are detailed below:
1. Defining the scope and architecture of the data platform
At the start of the project, it’s important to define clear requirements. This is where quality assurance steps in to ensure everything is well-documented and communicated.
The QA team helps by:
Clarifying objectives: making sure everyone understands the project’s goals.
Defining standards: setting the quality standards that the project must meet.
Identifying risks: highlighting potential issues that could affect the project’s success.
Organising review meetings: conducting architecture review meetings to address pending questions and identify potential risks.
Ensuring scalability: the platform needs to be able to handle growth and increased data loads.
Defining and agreeing on initial scope and key checks, including:
– The different raw data sources input from the landing to the bronze layer
– Rejected data storage and error logs for information not imported
– Failure process notifications and generating alerts
– Data cleaning and normalisation processes
– Record matching across different sources
– Data validations across the different layers
– Selecting the best information across sources to populate silver layer tables
2. Ensuring quality at each layer:
These platforms consist of several layers. To ensure that the processed data and outputs meet the required standards and are free from errors at each layer, quality assurance checks include:
- Check output data is correctly generated and stored.
- Verify that stored information is accurate and consistent. This involves checking for errors, duplicates, and inconsistencies that could compromise its quality.
- Ensure that data transformation steps are properly implemented. This includes verifying that the information is cleaned, normalised, and loaded correctly into the appropriate layers of the medallion architecture.
- The entire process should be efficiently executed, from data collection to the gold layer.
- Ensure that historical information is retained for future reference and compliance purposes at each layer.
3. Reporting (example with Power BI)
Quality assurance helps maintain high standards in reporting. This involves several key checks, such as:
Accuracy: confirm that reports display figures correctly by comparing them with gold-layer figures. This may involve collaborating with the data team to develop SQL queries to ensure Power BI shows accurate figures.
Usability: ensure reports are easy to understand and use. This includes gathering feedback and raising tickets for necessary adjustments.
Performance: check report performance to ensure they load quickly and can handle large datasets.
4. Automated testing
Automated testing helps detect errors or bugs early in the development process. This reduces the time and effort needed for manual testing.
Moreover, it speeds up the QA process, allowing for more frequent and reliable testing cycles.
5. Data Security and compliance
Ensuring data handling practices comply with relevant regulations and standards is crucial.
This includes protecting sensitive information from breaches, for instance, by using secure access controls.
Put quality assurance at the heart of your projects
Many consider data the new gold. As a valuable asset, companies must ensure the success of Big Data and Data Platform projects by empowering IT teams with the best resources, developing strong QA processes, and eventually considering support from external QA consultancies.
Furthermore, in these projects, quality assurance is not just a supporting role but a vital part of the process. QA ensures the integrity and success of the entire project by setting clear requirements, designing robust architectures, maintaining high data quality, and ensuring reports are accurate and user-friendly.
This makes quality assurance the foundation of any data platform project and essential for any organisation aiming to fully leverage the power of big data.
About the author: Dr Asma Zoghlami gained a PhD in computer science from the University Paris 8 (France) in 2013. Their research focused on the intersection of three disciplines AI, computer science and geomatics. They are currently a Test Lead at Acutest.